Artificial Intelligence (AI) Governance: Striking Balance Between Innovation and Ethical Considerations
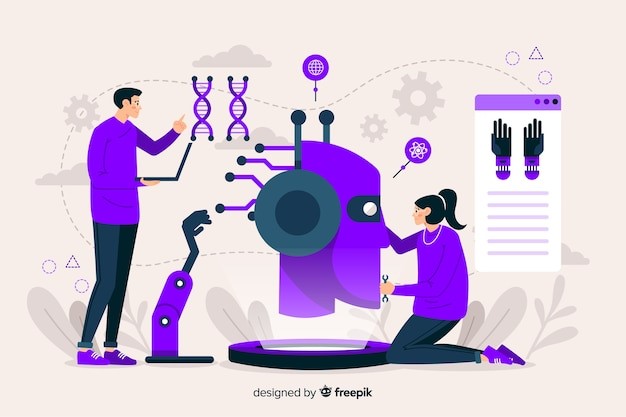
With the advent of generative AI, the search experience undergoes a revolutionary transformation. Instead of presenting a list of links to numerous articles, users now receive direct answers that are synthesized from a vast pool of data. Engaging with this technology is akin to having a conversation with an exceptionally intelligent machine. Similar technological advancements have been flourishing in the financial landscape as well. From fraud detection to credit score monitoring to providing personalized recommendations, AI models hold immense significance in the finance industry. Bloomberg LP has harnessed the power of AI technology, similar to OpenAI’s GPT trained on large financial data, to create its own AI model known as “Bloomberg GPT”. The company states Bloomberg GPT, its internal AI model, can more accurately answer questions like “CEO of Citigroup Inc?”, assess whether headlines are bearish or bullish for investors, and even write headlines based on short blurbs.
When it comes to using artificial intelligence (AI) solutions for business applications, it’s important to keep in mind that these solutions rely on non-deterministic algorithms. This means that they can’t be completely trusted without proper safeguards in place during both their development and implementation. Arising the need for AI Governance throughout the data lifecycle, specifically for financial institutions, as it may result in hefty penalties and reputational harm.
According to a recent report from independent intelligence platform Evident, banks across North America and Europe are failing to publicly report on their approaches to responsible AI development. Its research found that eight of the 23 largest banks in the US, Canada, and Europe currently provide no public responsible AI principles.” -The Banker
Need for Artificial Intelligence (AI) Governance
The practice of AI governance involves overseeing an organization’s use of artificial intelligence (AI). This includes keeping records of where the data and models used in artificial intelligence (AI) come from, as well as documenting the processes used to ensure transparency. The goal of AI governance is to provide insight into how models behave over time, including the methods used to train them, the metrics used to test them, and any potential risks to the organization. It also involves ongoing monitoring to ensure that models are fair, of high quality, and are not drifting from their intended purpose. One of the essential aspects of AI governance is providing visibility into the behavior of artificial intelligence (AI) models throughout the AI lifecycle.
Moreover, AI governance takes proactive measures to identify and validate potential risks before implementing a model into the business workflow. By thoroughly assessing AI applications’ ethical implications and potential biases, organizations can ensure the responsible and trustworthy use of AI technology.
After an artificial intelligence (AI) model goes live, AI governance remains vigilant by continuously monitoring its performance. This ongoing scrutiny allows for the detection of any unintended consequences or undesirable behaviors, such as biases or performance degradation over time. By identifying and addressing these issues promptly, organizations can maintain the quality and fairness of their artificial intelligence (AI) systems, preserving their integrity and reputation. Additionally, AI governance often involves collaboration between various stakeholders, including data scientists, CDOs, CIOs, financial officers, legal experts, and ethicists. By fostering multidisciplinary dialogue and cooperation, organizations can collectively address complex AI challenges.
Moreover, as discussions around the EU’s AI Act are advancing, various entities, governments, civil society, and businesses, are actively involved in guiding the advancement of artificial intelligence. The U.S. technology giants have also made commitments to collaborate with the White House in addressing and mitigating risks associated with artificial intelligence (AI) implementation.
The maximum fine that could be imposed under the proposed EU AI Act is EUR 30 million or, in the case of companies, 6 percent of their worldwide annual turnover, whichever is higher.- Source
By having a robust AI governance framework in place, organizations can instill accountability, responsibility, and oversight throughout the AI development and deployment process. This, in turn, fosters ethical and transparent AI practices, enhancing trust among users, customers, and the public.
Building Artificial Intelligence (AI) Governance Framework
The process begins with examining frameworks, knowing data challenges, and the process for reporting. The Chief Data Officers (CDOs) play a crucial role in ensuring artificial intelligence (AI) governance and data ethics within their organizations. Here are some key steps they can take to achieve this:
Establish Ethical Data Guidelines
CDOs should work with cross-functional teams, including legal, compliance, and data science, to develop clear and comprehensive ethical artificial intelligence (AI) guidelines. These guidelines should outline the principles and values that govern artificial intelligence (AI) implementation, addressing issues like privacy, fairness, transparency, and accountability.
Educate Stakeholders
CDOs should conduct training sessions and awareness programs for employees involved in data collection, processing, and artificial intelligence (AI) development. This helps ensure that all stakeholders understand the importance of data ethics and AI governance and their role in upholding these principles.
Implement Data Governance Frameworks
Implement robust data governance frameworks that include data classification, access controls, and data lifecycle management. This ensures that data is handled appropriately, respecting privacy and security requirements.
Risk Assessments
Conduct risk assessments to identify potential ethical issues and biases associated with artificial intelligence (AI) algorithms and data usage. Addressing these risks proactively can prevent negative consequences and reinforce trust in artificial intelligence (AI) systems.
Audit Artificial Intelligence (AI) Models
Regularly audit artificial intelligence (AI) models and data pipelines to identify and mitigate biases and other ethical concerns. Establish a feedback loop to continuously improve the models and ensure fairness and accuracy.
Promote Explainability
Encourage the use of interpretable artificial intelligence (AI) models that provide explanations for their decisions. This enhances transparency and helps build trust with customers and regulators.
Collaborate with Legal and Compliance Teams
Work closely with legal and compliance teams to ensure that artificial intelligence (AI) initiatives comply with relevant laws and regulations. This collaboration ensures that data processing and artificial intelligence (AI) implementation meet the required standards.
Encourage Responsible Artificial Intelligence (AI) Research
Foster a culture of responsible artificial intelligence (AI) research within the organization. Encourage data scientists and researchers to consider ethical implications when designing AI models and experiments.
Monitor and Review
Continuously monitor the performance and impact of artificial intelligence (AI) systems on various stakeholders, including customers and employees. Regularly review artificial intelligence (AI) -)-related processes and decisions to ensure they align with ethical standards.
Data Anonymization and Aggregation
Implement techniques like data anonymization and aggregation to protect the privacy of individuals while still utilizing valuable data for artificial intelligence (AI) purposes.
Why Consider Automating Artificial Intelligence (AI) Governance?
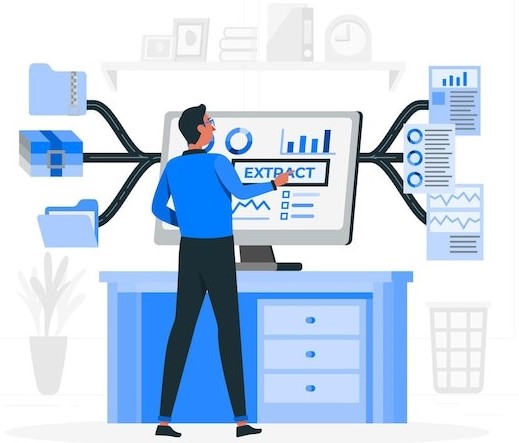
Using manual procedures for data validation and comparison in artificial intelligence (AI) governance can cause delays, and errors, and require costly expertise. Model validators may need to learn about each algorithm used, which can be time-consuming. Automating AI governance documentation and validation processes can greatly enhance efficiency and help companies avoid lagging behind competitors or missing auditor deadlines. Automation can enhance the efficiency and effectiveness of companies in integrating governance frameworks at the enterprise level.
How HEXANIKA can help you?
HEXANIKA’s data management platform #SmartJoin can help CDOs establish robust data governance frameworks. By ensuring data quality, accuracy, and consistency, CDOs can lay the foundation for ethical data practices and reliable artificial intelligence (AI) outcomes. The platform also provides data lineage capabilities, allowing tracking of the origin and transformation of data throughout its lifecycle. This promotes transparency, helping organizations understand how data is used in artificial intelligence (AI) models and decision-making processes. Our services also offer compliance with relevant regulatory regulations. This minimizes the risk of non-compliance and potential legal and reputational issues. Automate your processes to gain a competitive edge, Contact us at marketing@hexanika.com.